Enhancing Data Transformation with AI: A Standard Approach for Actionable Insights
- DKC Career
- Jan 9
- 4 min read
Updated: Jan 9
In today’s fast-paced world, organizations face an overwhelming flow of data from diverse sources. To thrive, transforming this data into actionable insights is essential for making informed decisions. The fusion of artificial intelligence (AI) and machine learning (ML) into the data transformation process serves as a game-changing strategy, enabling businesses to unlock the full potential of their analytics.
As competition heightens, mastering AI’s effective application can significantly enhance the value derived from data. This blog post explores how AI can revolutionize data transformation, yielding insights that drive positive change.
Understanding Data Transformation
Data transformation involves converting data from its original format into a more suitable format for analysis. This includes various stages such as data cleansing, normalization, and aggregation, all focused on enhancing data quality and accessibility.
The primary goal is to prepare data for meaningful analysis. In a world where over 2.5 quintillion bytes of data are generated daily, traditional methods alone are insufficient.
AI can accelerate data transformation processes, surpassing the capabilities of conventional techniques.
The Role of AI in Data Transformation

AI technologies, particularly through machine learning, utilize advanced algorithms to process and analyze data at astonishing speeds.
For instance, a retail company employing ML models noticed a 30% improvement in forecasting sales trends based on historical data. These models learn from past patterns, enabling predictive analytics that foster better decision-making. With AI integrated into your data strategy, organizations can quickly identify trends, uncover hidden insights, and accurately predict future outcomes.
This predictive ability equips businesses to respond promptly to market changes, enhancing overall agility.
Key Benefits of Integrating AI with Data Transformation
1. Improved Efficiency and Speed
AI-driven data transformation drastically shortens the time required for data preparation. Automation accelerates the cleansing, processing, and analysis of extensive datasets, allowing data engineers to concentrate on strategic endeavors.
In practice, organizations that adopted AI saw a 40% decrease in data preparation time, leading to quicker access to insights and timely decision-making.
2. Enhanced Accuracy and Consistency
Human error is a frequent challenge in traditional data transformation processes. AI minimizes this risk through automated data entry and verification, ensuring high accuracy and consistency.
For example, companies using AI-powered solutions reported a 25% reduction in errors, which translates into more reliable analytics and meaningful insights.
3. Scalability
As the volume of data continues to skyrocket, scalability is vital. AI systems can seamlessly scale to manage increasing data complexity and size, enabling organizations to continuously extract insights without disruption.
This adaptability ensures that as companies grow, their data analytics processes evolve in tandem.
4. Insightful Pattern Recognition
AI excels at recognizing intricate patterns that might remain unnoticed through manual examination. By implementing sophisticated algorithms, organizations can uncover trends, correlations, and anomalies in their data.
Such insights contribute to more informed and impactful business decisions, maximizing the return on data investment.
Implementing AI in Data Transformation: A Step-by-Step Approach
Step 1: Define Your Objectives
Before starting AI implementation, clearly establish the objectives of your data transformation initiatives. Identify specific goals, whether they focus on enhancing customer satisfaction, boosting operational efficiency, or improving analytics.
Having well-defined objectives will guide your AI strategy.
Step 2: Select the Right Tools and Technologies
Choose AI tools and platforms that align with your goals and data requirements. With numerous AI frameworks available, it's essential to select the ones best suited for your organization.
Conduct thorough research and consider running pilot tests to find the right fit before committing.
Step 3: Data Preparation
Ensure your data is clean, consistent, and structured. Quality data input is crucial for achieving high-quality output.
Implement standardized processes for data collection, storage, and management which facilitate smoother transformations. Organizations that prioritize data quality often see a 50% increase in actionable insights.
Step 4: Model Training and Evaluation
After preparing your data, train your AI models on historical datasets. This process helps the AI understand patterns and relationships within the data.
Regularly evaluate your models' performance and accuracy to ensure they meet your expectations. Anticipate the need for refinements along the way to maintain effectiveness.
Step 5: Implementation and Monitoring
Once trained, integrate your AI models into your data transformation processes. Continuous monitoring is necessary to ensure insights generated are relevant and actionable.
Establish feedback loops to improve the AI system based on real-world applications and outcomes.
Challenges in Data Transformation using AI
Despite its advantages, integrating AI into data transformation comes with challenges:
Data Quality Issues: Low-quality data can hinder AI performance. Address data hygiene upfront to avoid complications.
Skill Gaps: Implementing AI requires specialized expertise that may not be readily available. Organizations might need to upskill existing staff or bring in external specialists.
Cost Considerations: Implementing AI can involve significant upfront costs. A thorough cost-benefit analysis is critical to assess potential returns on investment.
Change Management: Resistance to change can be a barrier. Clearly communicating the benefits of AI and data transformation is vital for encouraging adoption.
Transforming Insights into Action
The ability to transform data into actionable insights is more important than ever in today’s data-driven environment. By incorporating AI and machine learning into data transformation processes, organizations can significantly enhance the speed, efficiency, and accuracy of their analytics.
A thoughtful, step-by-step approach to AI implementation can help overcome common obstacles, empowering data engineers to unearth deeper insights that drive meaningful change.
Ultimately, leveraging AI for data transformation not only strengthens decision-making but also positions organizations for a bright, data-centric future.
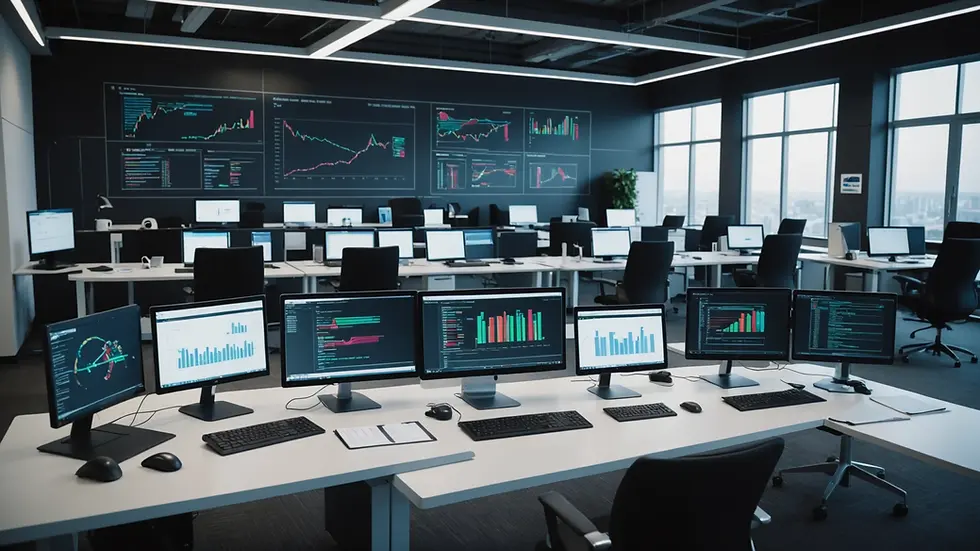
Comments